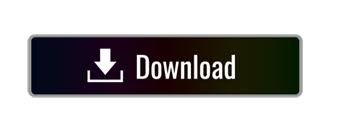
Since the Output Variable contains three classes (A, B, and C) to denote the three different wineries, the options for Classes in the Output Variable are disabled. Select a cell on the newly created Data_Partition worksheet, then on the XLMiner ribbon, from the Data Mining tab, select Classify - Neural Network - Boosting to open the Boosting - Neural Network Classification - Step 1 of 3 dialog.Īt Output Variable, select Type, and from the Selected Variables list, select all the remaining variables. For more information on partitioning a data set, see the Data Mining Partition section. Partition the data into Training and Validation Sets using a Standard Data Partition with percentages of 80% of the data randomly allocated to the Training Set, and 20% of the data randomly allocated to the Validation Set. On the XLMiner ribbon, from the Data Mining tab, select Partition - Standard Partition to open the Standard Data Partition dialog. A portion of this data set is shown below. The objective is to assign a wine classification to each record. This file contains 13 quantitative variables measuring the chemical attributes of wine samples from three different wineries (Type variable). On the XLMiner ribbon, from the Applying Your Model tab, select Help - Examples, then select Forecasting/Data Mining Examples to open the file Wine.xlsx.
#FRONTLINE SOLVER ANALYSIS ADDITION MANUAL#
See the sections below for examples of creating a Neural Network using the bagging ensemble method, a manual architecture, and an automatic architecture. This example focuses on creating a Neural Network using the boosting ensemble method. XLMiner provides four options when creating a Neural Network classifier: Boosting & Bagging (ensemble methods), Automatic, and Manual.
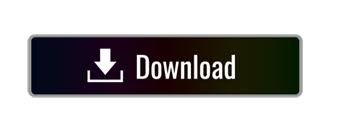